The Role of Statistics in Climate Research
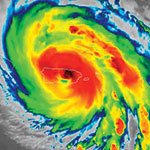
Image courtesy of NOAA. NOAA’s GOES16 satellite captured this colorized-infrared imagery of Hurricane Maria over Puerto Rico on September 20, 2017.
There is much discussion today about the role that the climate system plays in many aspects of our lives. Part of this conversation revolves around humanity’s interest in quantifying the state of the climate system now and in the future (understanding the science). The other part of the conversation involves assessing the impact of climate change, while potentially ascertaining reasons for the change.
The climate system is a complicated beast, involving the interaction of many physical processes that are changing over the air, land, sea, and ice-sheet. It has been long known that our climate system is chaotic—a nonlinear dynamical system—that can be hard to understand. Even simplified climate models give rise to multimodal relationships among variables, requiring careful analysis. Thus, in climate science, it can be difficult to quantify, with certainty, the current state of the system.
Statistical tools are required to assess, with uncertainty, what is currently known and where the system may be headed. Adding to the challenge, climate science, like many disciplines, is experiencing a data explosion. Satellites are observing the globe in increasing detail. Sophisticated climate models are producing model output on finer and finer spatial scales. Statisticians and new statistical techniques are required to use these data sources to improve our understanding of the current climate system and what it may look like in the future.
Recent and ongoing statistical research is creating new methodologies that can help to better answer open questions in climate science. Statisticians are developing methodologies to model large spatio-temporal data sets. They are building techniques to quantify and account for model discrepancy and to combine results from multiple models. New methods are being developed for modeling extremes and identifying changepoints. Hierarchical statistical modeling methods are able to blend physical and statistical models for improved statistical inference. Computer model output can be calibrated using statistical models.
This special issue of CHANCE draws together a wide range of articles that introduce how statistics can be used in climate science. Guttorp demonstrates, using time series analysis, how we model and assess global temperatures using observations, and compares these measurements to historical climate model simulations. Li and I extend these statistical comparisons to include spatio-temporal data from paleoclimate proxies, as well as instrumental data and climate model simulations. Jun introduces, more formally, what a global climate model is, and demonstrates how we learn about the commonalities and differences between climate model simulations using statistical tools.
The rest of the special issue starts to look at learning about climate impacts. Hammerling introduces the area of detection and attribution, usually carried out using counterfactual simulations of the climate system, to explain the possible factors causing climate change. Gilleland, Katz, and Naveau set forth the statistical science underlying the modeling of climate extremes. Haran, Chang, Keller, Nicholas, and Pollard communicate the science of ice sheets, and demonstrate how statisticians are able to work with scientists to predict future features of the Antarctic ice sheet. Wikle shows how statistical methods can be used to assess the synchronicity between the climate system and different ecological systems; and Chang, Sarnat, and Liu tell the story of predicting health effects under differing projections of the climate system.
A goal of this special issue is to encourage statisticians and climate scientists to work together on the hard problems that are at the interface of the two disciplines. As data sets and climate models become more complex, the challenges of carrying out climate research in small teams increase.
There is a need to build interdisciplinary research teams, bringing together varied skill sets, that can be used to solve important problems in climate science. It is necessary to understand what expertise each community can bring to working on interdisciplinary problems in climate science, but also to learn what skills each community needs to develop to be successful.
Both disciplines also could consider how each could train students and young researchers to work at the disciplines’ intersection.
This special issue was organized by the ASA’s Advisory Committee on Climate Change Policy, and several of the authors are either current or past members of this committee. The role of this committee is to “advise Congress on climate change issues, with special emphasis on the roles of statistics and statisticians in advancing science and informing policy.” Further details about the work of this committee can be found at the committee website.
Further Reading
Berliner, L.M. 2003. Uncertainty and climate change. Statistical Science 18, 430–435.
Katz, R.W., Craigmile, P.F., Guttorp, P., Haran, M., Sanso, B., and Stein, M.L. 2013. Uncertainty analysis in climate change assessments. Nature Climate Change 3, 769–771.
Sanso, B., Berliner, L.M., Cooley, D.S., Craigmile, P., Cressie, N.A., Haran, M., Lund, R.B., Nychka, D.W., Paciorek, C., Sain, S.R., Smith, R.L., and Stein, M.L. 2014. Statistical Science: Contributions to the Administrations Research Priority on Climate Change (PDF download). A White Paper of the American Statistical Associations Advisory Committee for Climate Change Policy.
Core Writing Team, Pachauri, R.K., and Meyer, L.A. (Eds.). Intergovernmental Panel on Climate Change. 2014. Climate Change 2014: Synthesis Report. Contribution of Working Groups I, II, and III to the Fifth Assessment Report of the Intergovernmental Panel on Climate Change. Geneva, Switzerland: IPCC.
Program on Mathematical and Statistical Methods for Climate and the Earth System. 2017–18. Durham, NC: Statistical and Applied Mathematical Sciences Institute.
About the Author
Peter F. Craigmile is a professor of statistics at The Ohio State University in Columbus, Ohio. In addition to other research interests, he likes to develop statistical methodology for analyzing data collected over space and time, which has application to modeling climate. He has served as chair of the ASA’s Advisory Committee on Climate Change Policy.
Any investigator, whether statistician, scientist, mathematician, or whoever, is free to define words without limitation, but define them he must if his contribution is to be rational. Chaos, nonlinear, systems, and dynamic systems, for example, could be stipulated so that the “climate system” would have those properties, comprising facts that could constitute knowledge. But as Lorenz used those terms, sometimes overlooking definitional rigor, they are properties of equations. His chaotic systems were models of the Real World where the models were expressed in equations. Nonetheless, Lorenz (1991) declared, “The atmosphere and its surroundings constitute a chaotic dynamic system”. However, sets comprised of coordinate systems and dimensions, variables and parameters, values and numbers, measures and units, clocks and scales, graphs and equations, logic and uncertainty are completely manmade. No such items exist in the Real World the thing that gives rise to man’s observations and measurements. What constitutes any “system” is an arbitrary human selection of variables. Failure to predict is a failure of the modeler, not a limitation on the Real World. Blaming the Real World is an excuse, not knowledge. The ability to distinguish between the Real World and a model of a portion of it is a basic concept in scientific literacy, and is a reason for teaching science.